A few weeks ago, I started digging into the FDA’s drug event dataset—curious to see what insights I could uncover. It didn’t take long to hit a wall. Healthcare datasets like this one come with a hidden performance cost: Moderate to high cardinality nested fields buried in JSON.
Take pharm_class_epc as an example—each record can include multiple pharmaceutical classes, and if you store that naively, you end up with loads of duplication. Worse still, your queries end up scanning everything.
I tackled this in DuckDB by applying a bit of classic relational thinking—normalizing the data and indexing those repeated fields. That turned a messy, unstructured sprawl into something far leaner: low-cardinality lookups that actually ran. It went from unusable to instant results.
Here’s how I pulled it off—and why cardinality turned out to be the key.
(Link to my original blog post)
The problem
The FDA drug event dataset is made up of millions of individual records stored as raw JSON. When downloaded, it’s around 480GB in size (at the time of writing)—a massive collection of deeply nested, semi-structured data. Raw JSON in DuckDB struggles with repeated nested fields, such as pharm_class_epc
, where the same strings appear across millions of records.. You end up with thousands of unique values repeated again and again across those millions of records. It’s a nightmare for storage—and worse for performance. You can’t index it properly, so even simple GROUP BYs turn into full scans that drag on for hours.
Here’s the “before” query I was running:
-- Top 10 most common pharm_class_epc values (from nested JSON)
SELECT
json_data->'openfda'->'pharm_class_epc' AS pharm_class_epc,
COUNT(*) AS count
FROM fda_drug_events
WHERE json_data->'openfda'->'pharm_class_epc' IS NOT NULL
GROUP BY json_data->'openfda'->'pharm_class_epc'
ORDER BY count DESC
LIMIT 10;
In case you’re wondering how bad it gets, here’s a screenshot of macOS warning me that PyCharm was using 62.52 GB of memory—just from running the “before” query in the DuckDB console.
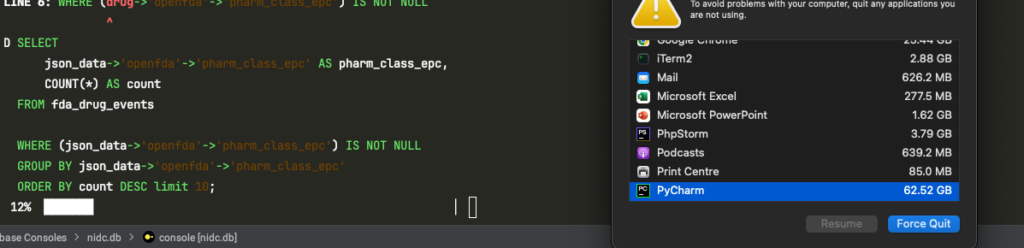
The solution
DuckDB doesn’t magically fix messy JSON—but once you flatten and normalize those moderate to high-cardinality fields, it really starts to shine. I pulled repeated values like pharm_class_epc into a separate lookup table with integer IDs, which gave me:
- Compact joins: Replacing strings with integers made the structure much leaner.
- Efficient scans: Queries now work on tight, flat tables—not nested arrays.
- Better scaling: Adding new classes doesn’t bloat the main dataset.
To make that work, I first had to extract the pharm_class_epc
values from the nested JSON and turn them into something relational. Instead of storing the same strings over and over, I built a dictionary table to hold unique values and assigned each one a numeric ID. Then I created a separate table to map each drug event to its corresponding classes using those IDs. That gave me a clean, normalized schema that plays to DuckDB’s strengths.
-- Lookup table for unique pharm_class_epc strings
CREATE TABLE IF NOT EXISTS pharm_class_epc_dict (
pharm_class_epc VARCHAR PRIMARY KEY,
pharm_class_epc_id INTEGER DEFAULT nextval('pharm_class_epc_dict_seq')
);
-- Expanded table linking drug event to pharm_class_epc_id
CREATE TABLE IF NOT EXISTS pharm_class_epc_expanded (
id BIGINT,
pharm_class_epc_id BIGINT
);
Here’s the function I used during import to look up or insert each pharm_class_epc
string:
def get_pharm_class_epc_id(conn, pharm_class_epc):
result = conn.execute("SELECT pharm_class_epc_id FROM pharm_class_epc_dict WHERE pharm_class_epc = ?", [pharm_class_epc]).fetchone()
if result:
return result[0]
else:
return conn.execute("INSERT INTO pharm_class_epc_dict (pharm_class_epc) VALUES (?) RETURNING pharm_class_epc_id", [pharm_class_epc]).fetchone()[0]
And a simplified snippet showing how that gets called when processing JSON:
for drug in json_data.get("patient", {}).get("drug", []):
pharm_class_epc_list = drug.get("openfda", {}).get("pharm_class_epc", [])
for pharm_class_epc in pharm_class_epc_list:
pharm_class_epc_id = get_pharm_class_epc_id(conn, pharm_class_epc)
# Insert or process pharm_class_epc_id as needed
Now that I’ve flattened things out and pushed pharm_class_epc
into its own lookup table, I can finally run that “after” query without the laptop sounding like it’s about to lift off. No more 60GB memory spikes or force-quitting PyCharm mid-query. Instead, the same aggregation now completes in just 0.166 seconds real time.
SELECT d.pharm_class_epc, COUNT(*) AS count
FROM pharm_class_epc_expanded e
JOIN pharm_class_epc_dict d ON e.pharm_class_epc_id = d.pharm_class_epc_id
GROUP BY d.pharm_class_epc
ORDER BY count DESC
LIMIT 10;
That’s not a typo
From beachballing to blink-of-an-eye — all thanks to a bit of normalization.
Here’s a screenshot of that same query completing successfully in the terminal:
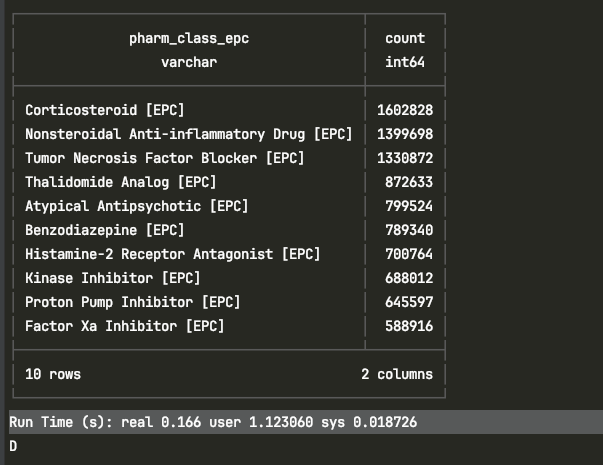
This shows the “after” query executing in under a second, using the normalized schema with indexed integer lookups.
Wrapping up
This was a small but satisfying win—and a great reminder that sometimes the answer isn’t more compute or bigger machines, it’s just better structure.
If you’re interested in how I’m using data and generative AI to help tackle polypharmacy, you can find out more at datasignal.uk.